Mixed-Radix Cooley-Tukey FFT
When the desired DFT length can be expressed as a product of
smaller integers, the Cooley-Tukey decomposition provides what is
called a mixed radix Cooley-Tukey FFT algorithm.A.2
Two basic varieties of Cooley-Tukey FFT are decimation in time (DIT) and its Fourier dual, decimation in frequency (DIF). The next section illustrates decimation in time.
Decimation in Time
The DFT is defined by





When is even, the DFT summation can be split into sums over the
odd and even indexes of the input signal:
where
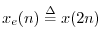







Radix 2 FFT
When is a power of
, say
where
is an integer,
then the above DIT decomposition can be performed
times, until
each DFT is length
. A length
DFT requires no multiplies. The
overall result is called a radix 2 FFT. A different radix 2
FFT is derived by performing decimation in frequency.
A split radix FFT is theoretically more efficient than a pure radix 2 algorithm [73,31] because it minimizes real arithmetic operations. The term ``split radix'' refers to a DIT decomposition that combines portions of one radix 2 and two radix 4 FFTs [22].A.3On modern general-purpose processors, however, computation time is often not minimized by minimizing the arithmetic operation count (see §A.7 below).
Radix 2 FFT Complexity is N Log N
Putting together the length DFT from the
length-
DFTs in
a radix-2 FFT, the only multiplies needed are those used to combine
two small DFTs to make a DFT twice as long, as in Eq.
(A.1).
Since there are approximately
(complex) multiplies needed for each
stage of the DIT decomposition, and only
stages of DIT (where
denotes the log-base-2 of
), we see that the total number
of multiplies for a length
DFT is reduced from
to
, where
means ``on the order of
''. More
precisely, a complexity of
means that given any
implementation of a length-
radix-2 FFT, there exist a constant
and integer
such that the computational complexity
satisfies
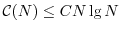


Fixed-Point FFTs and NFFTs
Recall (e.g., from Eq.(6.1)) that the inverse DFT requires a
division by
that the forward DFT does not. In fixed-point
arithmetic (Appendix G), and when
is a power of 2,
dividing by
may be carried out by right-shifting
places in the binary word. Fixed-point implementations of the inverse
Fast Fourier Transforms (FFT) (Appendix A) typically right-shift one
place after each Butterfly stage. However, superior overall numerical
performance may be obtained by right-shifting after every other
butterfly stage [8], which corresponds to dividing both the
forward and inverse FFT by
(i.e.,
is implemented
by half as many right shifts as dividing by
). Thus, in
fixed-point, numerical performance can be improved, no extra work is
required, and the normalization work (right-shifting) is spread
equally between the forward and reverse transform, instead of
concentrating all
right-shifts in the inverse transform. The NDFT
is therefore quite attractive for fixed-point implementations.
Because signal amplitude can grow by a factor of 2 from one butterfly
stage to the next, an extra guard bit is needed for each pair of
subsequent NDFT butterfly stages. Also note that if the DFT length
is not a power of
, the number of right-shifts in the
forward and reverse transform must differ by one (because
is odd instead of even).
Next Section:
Prime Factor Algorithm (PFA)
Previous Section:
Recommended Further Reading