
Closing the gap: CPU and FPGA Trends in sustainable floating-point BLAS performance
Field programmable gate arrays (FPGAs) have long been an attractive alternative to microprocessors for computing tasks — as long as floating-point arithmetic is not required. Fueled by the advance of Moore’s Law, FPGAs are rapidly reaching sufficient densities to enhance peak floating-point performance as well. The question, however, is how much of this peak performance can be sustained. This paper examines three of the basic linear algebra subroutine (BLAS) functions: vector dot product, matrix-vector multiply, and matrix multiply. A comparison of microprocessors, FPGAs, and Reconfigurable Computing platforms is performed for each operation. The analysis highlights the amount of memory bandwidth and internal storage needed to sustain peak performance with FPGAs. This analysis considers the historical context of the last six years and is extrapolated for the next six years.

BLAS Comparison on FPGA, CPU and GPU
High Performance Computing (HPC) or scientific codes are being executed across a wide variety of computing platforms from embedded processors to massively parallel GPUs. We present a comparison of the Basic Linear Algebra Subroutines (BLAS) using double-precision floating point on an FPGA, CPU and GPU. On the CPU and GPU, we utilize standard libraries on state-of-the-art devices. On the FPGA, we have developed parameterized modular implementations for the dot product and Gaxpy or matrix-vector multiplication. In order to obtain optimal performance for any aspect ratio of the matrices, we have designed a high-throughput accumulator to perform an efficient reduction of floating point values. To support scalability to large data-sets, we target the BEE3 FPGA platform. We use performance and energy efficiency as metrics to compare the different platforms. Results show that FPGAs offer comparable performance as well as 2.7 to 293 times better energy efficiency for the test cases that we implemented on all three platforms.
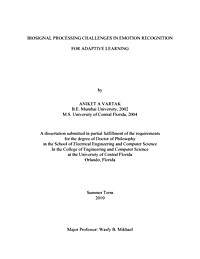
Biosignal processing challenges in emotion recognition for adaptive learning
User-centered computer based learning is an emerging field of interdisciplinary research. Research in diverse areas such as psychology, computer science, neuroscience and signal processing is making contributions to take this field to the next level. Learning systems built using contributions from these fields could be used in actual training and education instead of just laboratory proof-of-concept. One of the important advances in this research is the detection and assessment of the cognitive and emotional state of the learner using such systems. This capability moves development beyond the use of traditional user performance metrics to include system intelligence measures that are based on current theories in neuroscience. These advances are of paramount importance in the success and wide spread use of learning systems that are automated and intelligent. Emotion is considered an important aspect of how learning occurs, and yet estimating it and making adaptive adjustments are not part of most learning systems. In this research we focus on one specific aspect of constructing an adaptive and intelligent learning system, that is, estimation of the emotion of the learner as he/she is using the automated training system. The challenge starts with the definition of the emotion and the utility of it in human life. The next challenge is to measure the co-varying factors of the emotions in a non-invasive way, and find consistent features from these measures that are valid across wide population. In this research we use four physiological sensors that are non-invasive, and establish a methodology of utilizing the data from these sensors using different signal processing tools. A validated set of visual stimuli used worldwide in the research of emotion and attention, called International Affective Picture System (IAPS), is used. A dataset is collected from the sensors in an experiment designed to elicit emotions from these validated visual stimuli. We describe a novel wavelet method to calculate hemispheric asymmetry metric using electroencephalography data. This method is tested against typically used power spectral density method. We show overall improvement in accuracy in classifying specific emotions using the novel method. We also show distinctions between different discrete emotions from the autonomic nervous system activity using electrocardiography, electrodermal activity and pupil diameter changes. Findings from different features from these sensors are used to give guidelines to use each of the individual sensors in the adaptive learning environment.

Gauss-Newton Based Learning for Fully Recurrent Neural Networks
The thesis discusses a novel off-line and on-line learning approach for Fully Recurrent Neural Networks (FRNNs). The most popular algorithm for training FRNNs, the Real Time Recurrent Learning (RTRL) algorithm, employs the gradient descent technique for finding the optimum weight vectors in the recurrent neural network. Within the framework of the research presented, a new off-line and on-line variation of RTRL is presented, that is based on the Gauss-Newton method. The method itself is an approximate Newton’s method tailored to the specific optimization problem, (non-linear least squares), which aims to speed up the process of FRNN training. The new approach stands as a robust and effective compromise between the original gradient-based RTRL (low computational complexity, slow convergence) and Newton-based variants of RTRL (high computational complexity, fast convergence). By gathering information over time in order to form Gauss-Newton search vectors, the new learning algorithm, GN-RTRL, is capable of converging faster to a better quality solution than the original algorithm. Experimental results reflect these qualities of GN-RTRL, as well as the fact that GN-RTRL may have in practice lower computational cost in comparison, again, to the original RTRL.
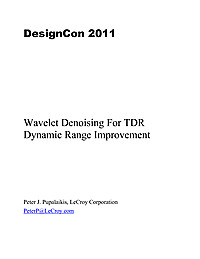
Wavelet Denoising for TDR Dynamic Range Improvement
A technique is presented for removing large amounts of noise present in time-domain-reflectometry (TDR) waveforms to increase the dynamic range of TDR waveforms and TDR based s-parameter measurements.
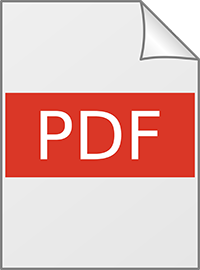
Bilinear Transformation Made Easy
A formula is derived and demonstrated that is capable of directly generating digital filter coefficients from an analog filter prototype using the bilinear transformation. This formula obviates the need for any algebraic manipulation of the analog prototype filter and is ideal for use in embedded systems that must take in any general analog filter specification and dynamically generate digital filter coefficients directly usable in difference equations.
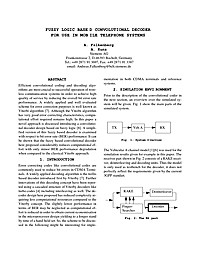
FUZZY LOGIC BASED CONVOLUTIONAL DECODER FOR USE IN MOBILE TELEPHONE SYSTEMS
Efficient convolutional coding and decoding algorithms are most crucial to successful operation of wireless communication systems in order to achieve high quality of service by reducing the overall bit error rate performance. A widely applied and well evaluated scheme for error correction purposes is well known as Viterbi algorithm [7]. Although the Viterbi algorithm has very good error correcting characteristics, computational effort required remains high. In this paper a novel approach is discussed introducing a convolutional decoder design based on fuzzy logic. A simplified version of this fuzzy based decoder is examined with respect to bit error rate (BER) performance. It can be shown that the fuzzy based convolutional decoder here proposed considerably reduces computational effort with only minor BER performance degradation when compared to the classical Viterbi approach.

Method to Calculate the Inverse of a Complex Matrix using Real Matrix Inversion
This paper describes a simple method to calculate the invers of a complex matrix. The key element of the method is to use a matrix inversion, which is available and optimised for real numbers. Some actual libraries used for digital signal processing only provide highly optimised methods to calculate the inverse of a real matrix, whereas no solution for complex matrices are available, like in [1]. The presented algorithm is very easy to implement, while still much more efficient than for example the method presented in [2]. [1] Visual DSP++ 4.0 C/C++ Compiler and Library Manual for TigerSHARC Processors; Analog Devices; 2005. [2] W. Press, S.A. Teukolsky, W.T. Vetterling, B.R. Flannery; Numerical Recipes in C++, The art of scientific computing, Second Edition; p52 : “Complex Systems of Equations”;Cambridge University Press 2002.
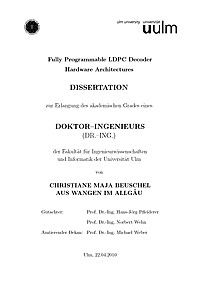
Fully Programmable LDPC Decoder Hardware Architectures
In recent years, the amount of digital data which is stored and transmitted for private and public usage has increased considerably. To allow a save transmission and storage of data despite of error-prone transmission media, error correcting codes are used. A large variety of codes has been developed, and in the past decade low-density parity-check (LDPC) codes which have an excellent error correction performance became more and more popular. Today, low-density parity-check codes have been adopted for several standards, and efficient decoder hardware architectures are known for the chosen structured codes. However, the existing decoder designs lack flexibility as only few structured codes can be decoded with one decoder chip. In consequence, different codes require a redesign of the decoder, and few solutions exist for decoding of codes which are not quasi-cyclic or which are unstructured. In this thesis, three different approaches are presented for the implementation of fully programmable LDPC decoders which can decode arbitrary LDPC codes. As a design study, the first programmable decoder which uses a heuristic mapping algorithm is realized on an field-programmable gate array (FPGA), and error correction curves are measured to verify the correct functionality. The main contribution of this thesis lies in the development of the second and the third architecture and an appropriate mapping algorithm. The proposed fully programmable decoder architectures use one-phase message passing and layered decoding and can decode arbitrary LDPC codes using an optimum mapping and scheduling algorithm. The presented programmable architectures are in fact generalized decoder architectures from which the known decoders architectures for structured LDPC codes can be derived.

Design of a Scalable Polyphony-MIDI Synthesizer for a Low Cost DSP
In this thesis, the design of a music synthesizer implementing the Scalable Polyphony-MIDI soundset on a low cost DSP system is presented. First, the SP-MIDI standard and the target DSP platform are presented followed by review of commonly used synthesis techniques and their applicability to systems with limited computational and memory resources. Next, various oscillator and filter algorithms used in digital subtractive synthesis are reviewed in detail. Special attention is given to the aliasing problem caused by discontinuities in classical waveforms, such as sawtooth and pulse waves and existing methods for bandlimited waveform synthesis are presented. This is followed by review of established structures for computationally efficient time-varying filters. A novel digital structure is presented that decouples the cutoff and resonance controls. The new structure is based on the analog Korg MS-20 lowpass filter and is computationally very efficient and well suited for implementation on low bitdepth architectures. Finally, implementation issues are discussed with emphasis on the Differentiated Parabole Wave oscillator and MS-20 filter structures and the effects of limited computational capability and low bitdepth. This is followed by designs for several example instruments.
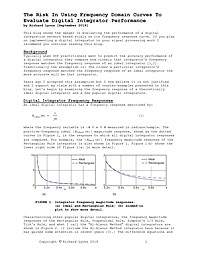
The Risk In Using Frequency Domain Curves To Evaluate Digital Integrator Performance
This article shows the danger in evaluating the performance of a digital integration network based solely on its frequency response curve. If you plan on implementing a digital integrator in your signal processing work I recommend you continue reading this article.
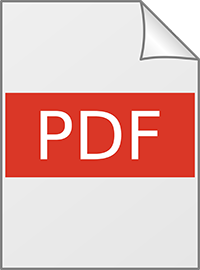
An Experimental Multichannel Pulse Code Modulation System of Toll Quality + Electron Beam Deflection Tube For Pulse Code Modulation
See this blog post for context. Pulse Code Modulation offers attractive possibilities for multiplex telephony via such media as the microwave radio relay. The various problems involved in its use have been explored in terms of a 96-channel system designed to meet the transmission requirements commonly imposed upon commercial toll circuits. Twenty-four of the 96 channels have been fully equipped in an experimental model of the system. Coding and decoding devices are described, along with other circuit details. The coder is based upon a new electron beam tube, and is characterized by speed and simplicity as well as accuracy of coding. These qualities are matched in the decoder, which employs pulse excitation of a simple reactive network.
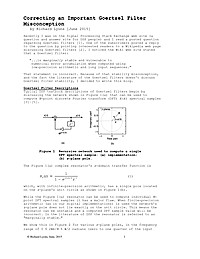
Correcting an Important Goertzel Filter Misconception
Correcting an Important Goertzel Filter Misconception
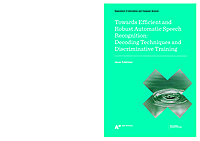
Towards Efficient and Robust Automatic Speech Recognition: Decoding Techniques and Discriminative Training
Automatic speech recognition has been widely studied and is already being applied in everyday use. Nevertheless, the recognition performance is still a bottleneck in many practical applications of large vocabulary continuous speech recognition. Either the recognition speed is not sufficient, or the errors in the recognition result limit the applications. This thesis studies two aspects of speech recognition, decoding and training of acoustic models, to improve speech recognition performance in different conditions.
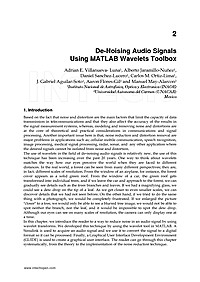
De-Noising Audio Signals Using MATLAB Wavelets Toolbox
Based on the fact that noise and distortion are the main factors that limit the capacity of data transmission in telecommunications and that they also affect the accuracy of the results in the signal measurement systems, whereas, modeling and removing noise and distortions are at the core of theoretical and practical considerations in communications and signal processing. Another important issue here is that, noise reduction and distortion removal are major problems in applications such as; cellular mobile communication, speech recognition, image processing, medical signal processing, radar, sonar, and any other application where the desired signals cannot be isolated from noise and distortion. The use of wavelets in the field of de-noising audio signals is relatively new, the use of this technique has been increasing over the past 20 years. One way to think about wavelets matches the way how our eyes perceive the world when they are faced to different distances. In the real world, a forest can be seen from many different perspectives; they are, in fact, different scales of resolution. From the window of an airplane, for instance, the forest cover appears as a solid green roof. From the window of a car, the green roof gets transformed into individual trees, and if we leave the car and approach to the forest, we can gradually see details such as the trees branches and leaves. If we had a magnifying glass, we could see a dew drop on the tip of a leaf. As we get closer to even smaller scales, we can discover details that we had not seen before. On the other hand, if we tried to do the same thing with a photograph, we would be completely frustrated. If we enlarged the picture "closer" to a tree, we would only be able to see a blurred tree image; we would not be able to spot neither the branch, nor the leaf, and it would be impossible to spot the dew drop. Although our eyes can see on many scales of resolution, the camera can only display one at a time. In this chapter, we introduce the reader to a way to reduce noise in an audio signal by using wavelet transforms. We developed this technique by using the wavelet tool in MATLAB. A Simulink is used to acquire an audio signal and we use it to convert the signal to a digital format so it can be processed. Finally, a Graphical User Interface Development Environment (GUIDE) is used to create a graphical user interface. The reader can go through this chapter systematically, from the theory to the implementation of the noise reduction technique. We will introduce in the first place the basic theory of an audio signal, the noise treatment fundamentals and principles of the wavelets theory. Then, we will present the development of noise reduction when using wavelet functions in MATLAB. In the foreground, we will demonstrate the usefulness of wavelets to reduce noise in a model system where Gaussian noise is inserted to an audio signal. In the following sections, we will present a practical example of noise reduction in a sinusoidal signal that has been generated in the MATLAB, which it is followed by an example with a real audio signal captured via Simulink. Finally, the graphic noise reduction model using GUIDE will be shown.

High speed data collection with Blackfin DSP
This report covers a master thesis in embedded systems, the goal of which was to investigate the high speed data collection capabilities with a Blackfin DSP. Basic theory about sampling and noise is covered briefly from a practical point of view. The theory is intended to be useful for those diving into a ADC datasheet for the first time. After an investigation of the delimiting factors, suitable components were selected and a prototype ADC PCB was designed from scratch. The goal is to design a general low noise data collecting unit compatible with the Blackfin DSP. Finally simple DSP software is designed to prove that DSP can handle such a high datastream.Testing the ADC card with the target Blackfin platform indicates thatthe analog parts indeed works. An analog bandwidth of over 10MHz ismeasured at a resolution exceeding 10 bits with respect to noise. The digital parts intended to interleave the two channels digital streams into one Blackfin unit did not work as intended. Only one channel is supported as of now. The report contains suggestions for future work in this area.
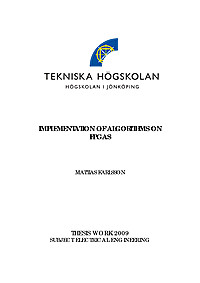
Implementation of Algorithms on FPGAs
This thesis describes how an algorithm is transferred from a digital signal processor to an embedded microprocessor in an FPGA using C to hardware program from Altera. Saab Avitronics develops the secondary high lift control system for the Boeing 787 aircraft. The high lift system consists of electric motors controlling the trailing edge wing flaps and the leading edge wing slats. The high lift motors manage to control the Boeing 787 aircraft with full power even if half of each motor’s stators are damaged. The motor is a PMDC brushless motor which is controlled by an advanced algorithm. The algorithm needs to be calculated by a fast special digital signal processor. In this thesis I have tested if the algorithm can be transferred to an FPGA and still manage the time and safety demands. This was done by transferring an already working algorithm from the digital signal processor to an FPGA. The idea was to put the algorithm in an embedded NIOS II microprocessor and speed up the bottlenecks with Altera’s C to hardware program. The study shows that the C-code needs to be optimized for C to hardware to manage the up speeding part, as the tests showed that the calculation time for the algorithm actually became longer with C to hardware. This thesis also shows that it is highly probable to use an FPGA equipped with Altera’s NIOS II safety critical microprocessor instead of a digital signal processor to control the electrical high lift motors in the Boeing 787 aircraft.
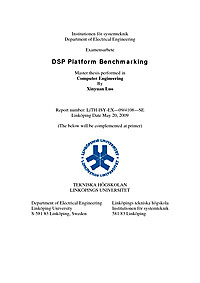
DSP Platform Benchmarking
Benchmarking of DSP kernel algorithms was conducted in the thesis on a DSP processor for teaching in the course TESA26 in the department of Electrical Engineering. It includes benchmarking on cycle count and memory usage. The goal of the thesis is to evaluate the quality of a single MAC DSP instruction set and provide suggestions for further improvement in instruction set architecture accordingly. The scope of the thesis is limited to benchmark the processor only based on assembly coding. The quality check of compiler is not included. The method of the benchmarking was proposed by BDTI, Berkeley Design Technology Incorporations, which is the general methodology used in world wide DSP industry. Proposals on assembly instruction set improvements include the enhancement of FFT and DCT. The cycle cost of the new FFT benchmark based on the proposal was XX% lower, showing that the proposal was right and qualified. Results also show that the proposal promotes the cycle cost score for matrix computing, especially matrix multiplication. The benchmark results were compared with general scores of single MAC DSP processors offered by BDTI.
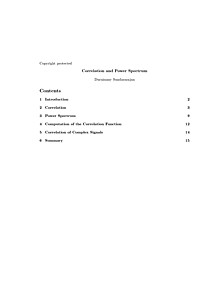
Correlation and Power Spectrum
In the signals and systems course and in the first course in digital signal processing, a signal is, most often, characterized by its amplitude spectrum in the frequency-domain and its amplitude profile in the time-domain. So much a student gets used to this type of characterization, that the student finds it difficult to appreciate, when encountered in the ensuing statistical signal processing course, the fact that a signal can also be characterized by its autocorrelation function in the time-domain and the corresponding power spectrum in the frequency-domain and that the amplitude characterization is not available. In this article, the characterization of a signal by its autocorrelation function in the time-domain and the corresponding power spectrum in the frequency-domain is described. Cross-correlation of two signals is also presented.