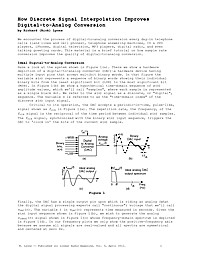
How Discrete Signal Interpolation Improves D/A Conversion
Earlier this year, for the Linear Audio magazine, published in the Netherlands whose subscribers are technically-skilled hi-fi audio enthusiasts, I wrote an article on the fundamentals of interpolation as it's used to improve the performance of analog-to-digital conversion. Perhaps that article will be of some value to the subscribers of dsprelated.com. Here's what I wrote: We encounter the process of digital-to-analog conversion every day—in telephone calls (land lines and cell phones), telephone answering machines, CD & DVD players, iPhones, digital television, MP3 players, digital radio, and even talking greeting cards. This material is a brief tutorial on how sample rate conversion improves the quality of digital-to-analog conversion.

Understanding the 'Phasing Method' of Single Sideband Demodulation
There are four ways to demodulate a transmitted single sideband (SSB) signal. Those four methods are: synchronous detection, phasing method, Weaver method, and filtering method. Here we review synchronous detection in preparation for explaining, in detail, how the phasing method works. This blog contains lots of preliminary information, so if you're already familiar with SSB signals you might want to scroll down to the 'SSB DEMODULATION BY SYNCHRONOUS DETECTION' section.
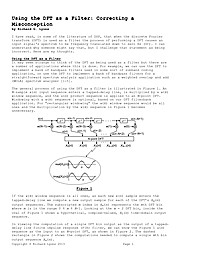
Using the DFT as a Filter: Correcting a Misconception
I have read, in some of the literature of DSP, that when the discrete Fourier transform (DFT) is used as a filter the process of performing a DFT causes an input signal's spectrum to be frequency translated down to zero Hz (DC). I can understand why someone might say that, but I challenge that statement as being incorrect. Here are my thoughts.

A Quadrature Signals Tutorial: Complex, But Not Complicated
Quadrature signals are based on the notion of complex numbers and perhaps no other topic causes more heartache for newcomers to DSP than these numbers and their strange terminology of j operator, complex, imaginary, real, and orthogonal. If you're a little unsure of the physical meaning of complex numbers and the j = √-1 operator, don't feel bad because you're in good company. Why even Karl Gauss, one the world's greatest mathematicians, called the j operator the "shadow of shadows". Here we'll shine some light on that shadow so you'll never have to call the Quadrature Signal Psychic Hotline for help. Quadrature signal processing is used in many fields of science and engineering, and quadrature signals are necessary to describe the processing and implementation that takes place in modern digital communications systems. In this tutorial we'll review the fundamentals of complex numbers and get comfortable with how they're used to represent quadrature signals. Next we examine the notion of negative frequency as it relates to quadrature signal algebraic notation, and learn to speak the language of quadrature processing. In addition, we'll use three-dimensional time and frequency-domain plots to give some physical meaning to quadrature signals. This tutorial concludes with a brief look at how a quadrature signal can be generated by means of quadrature-sampling.
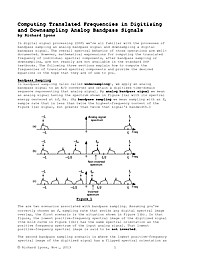
Computing Translated Frequencies in Digitizing and Downsampling Analog Bandpass Signals
In digital signal processing (DSP) we're all familiar with the processes of bandpass sampling an analog bandpass signal and downsampling a digital bandpass signal. The overall spectral behavior of those operations are well-documented. However, mathematical expressions for computing the translated frequency of individual spectral components, after bandpass sampling or downsampling, are not available in the standard DSP textbooks. This document explains how to compute the frequencies of translated spectral components and provide the desired equations in the hope that they are of use to you.
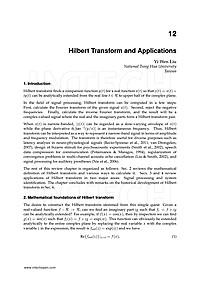
Hilbert Transform and Applications
Section 1: reviews the mathematical definition of Hilbert transform and various ways to calculate it.
Sections 2 and 3: review applications of Hilbert transform in two major areas: Signal processing and system identification.
Section 4: concludes with remarks on the historical development of Hilbert transform

Voice Activity Detection. Fundamentals and Speech Recognition System Robustness
An important drawback affecting most of the speech processing systems is the environmental noise and its harmful effect on the system performance. Examples of such systems are the new wireless communications voice services or digital hearing aid devices. In speech recognition, there are still technical barriers inhibiting such systems from meeting the demands of modern applications. Numerous noise reduction techniques have been developed to palliate the effect of the noise on the system performance and often require an estimate of the noise statistics obtained by means of a precise voice activity detector (VAD). Speech/non-speech detection is an unsolved problem in speech processing and affects numerous applications including robust speech recognition, discontinuous transmission, real-time speech transmission on the Internet or combined noise reduction and echo cancellation schemes in the context of telephony. The speech/non-speech classification task is not as trivial as it appears, and most of the VAD algorithms fail when the level of background noise increases. During the last decade, numerous researchers have developed different strategies for detecting speech on a noisy signal and have evaluated the influence of the VAD effectiveness on the performance of speech processing systems. Most of the approaches have focussed on the development of robust algorithms with special attention being paid to the derivation and study of noise robust features and decision rules. The different VAD methods include those based on energy thresholds, pitch detection, spectrum analysis, zero-crossing rate, periodicity measure, higher order statistics in the LPC residual domain or combinations of different features. This chapter shows a comprehensive approximation to the main challenges in voice activity detection, the different solutions that have been reported in a complete review of the state of the art and the evaluation frameworks that are normally used. The application of VADs for speech coding, speech enhancement and robust speech recognition systems is shown and discussed. Three different VAD methods are described and compared to standardized and recently reported strategies by assessing the speech/non-speech discrimination accuracy and the robustness of speech recognition systems.

Digital Image Processing Using LabView
Digital Image processing is a topic of great relevance for practically any project, either for basic arrays of photodetectors or complex robotic systems using artificial vision. It is an interesting topic that offers to multimodal systems the capacity to see and understand their environment in order to interact in a natural and more efficient way. The development of new equipment for high speed image acquisition and with higher resolutions requires a significant effort to develop techniques that process the images in a more efficient way. Besides, medical applications use new image modalities and need algorithms for the interpretation of these images as well as for the registration and fusion of the different modalities, so that the image processing is a productive area for the development of multidisciplinary applications. The aim of this chapter is to present different digital image processing algorithms using LabView and IMAQ vision toolbox. IMAQ vision toolbox presents a complete set of digital image processing and acquisition functions that improve the efficiency of the projects and reduce the programming effort of the users obtaining better results in shorter time. Therefore, the IMAQ vision toolbox of LabView is an interesting tool to analyze in detail and through this chapter it will be presented different theories about digital image processing and different applications in the field of image acquisition, image transformations. This chapter includes in first place the image acquisition and some of the most common operations that can be locally or globally applied, the statistical information generated by the image in a histogram is commented later. Finally, the use of tools allowing to segment or filtrate the image are described making special emphasis in the algorithms of pattern recognition and matching template.
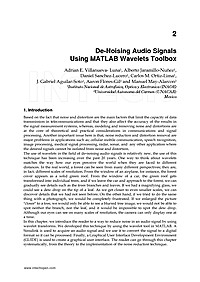
De-Noising Audio Signals Using MATLAB Wavelets Toolbox
Based on the fact that noise and distortion are the main factors that limit the capacity of data transmission in telecommunications and that they also affect the accuracy of the results in the signal measurement systems, whereas, modeling and removing noise and distortions are at the core of theoretical and practical considerations in communications and signal processing. Another important issue here is that, noise reduction and distortion removal are major problems in applications such as; cellular mobile communication, speech recognition, image processing, medical signal processing, radar, sonar, and any other application where the desired signals cannot be isolated from noise and distortion. The use of wavelets in the field of de-noising audio signals is relatively new, the use of this technique has been increasing over the past 20 years. One way to think about wavelets matches the way how our eyes perceive the world when they are faced to different distances. In the real world, a forest can be seen from many different perspectives; they are, in fact, different scales of resolution. From the window of an airplane, for instance, the forest cover appears as a solid green roof. From the window of a car, the green roof gets transformed into individual trees, and if we leave the car and approach to the forest, we can gradually see details such as the trees branches and leaves. If we had a magnifying glass, we could see a dew drop on the tip of a leaf. As we get closer to even smaller scales, we can discover details that we had not seen before. On the other hand, if we tried to do the same thing with a photograph, we would be completely frustrated. If we enlarged the picture "closer" to a tree, we would only be able to see a blurred tree image; we would not be able to spot neither the branch, nor the leaf, and it would be impossible to spot the dew drop. Although our eyes can see on many scales of resolution, the camera can only display one at a time. In this chapter, we introduce the reader to a way to reduce noise in an audio signal by using wavelet transforms. We developed this technique by using the wavelet tool in MATLAB. A Simulink is used to acquire an audio signal and we use it to convert the signal to a digital format so it can be processed. Finally, a Graphical User Interface Development Environment (GUIDE) is used to create a graphical user interface. The reader can go through this chapter systematically, from the theory to the implementation of the noise reduction technique. We will introduce in the first place the basic theory of an audio signal, the noise treatment fundamentals and principles of the wavelets theory. Then, we will present the development of noise reduction when using wavelet functions in MATLAB. In the foreground, we will demonstrate the usefulness of wavelets to reduce noise in a model system where Gaussian noise is inserted to an audio signal. In the following sections, we will present a practical example of noise reduction in a sinusoidal signal that has been generated in the MATLAB, which it is followed by an example with a real audio signal captured via Simulink. Finally, the graphic noise reduction model using GUIDE will be shown.
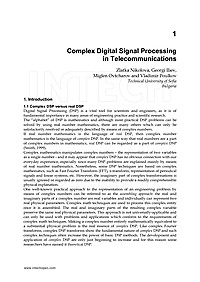
Complex Digital Signal Processing in Telecommunications
Digital Signal Processing (DSP) is a vital tool for scientists and engineers, as it is of fundamental importance in many areas of engineering practice and scientific research. The "alphabet" of DSP is mathematics and although most practical DSP problems can be solved by using real number mathematics, there are many others which can only be satisfactorily resolved or adequately described by means of complex numbers. If real number mathematics is the language of real DSP, then complex number mathematics is the language of complex DSP. In the same way that real numbers are a part of complex numbers in mathematics, real DSP can be regarded as a part of complex DSP (Smith, 1999). Complex mathematics manipulates complex numbers - the representation of two variables as a single number - and it may appear that complex DSP has no obvious connection with our everyday experience, especially since many DSP problems are explained mainly by means of real number mathematics. Nonetheless, some DSP techniques are based on complex mathematics, such as Fast Fourier Transform (FFT), z-transform, representation of periodical signals and linear systems, etc. However, the imaginary part of complex transformations is usually ignored or regarded as zero due to the inability to provide a readily comprehensible physical explanation. One well-known practical approach to the representation of an engineering problem by means of complex numbers can be referred to as the assembling approach: the real and imaginary parts of a complex number are real variables and individually can represent two real physical parameters. Complex math techniques are used to process this complex entity once it is assembled. The real and imaginary parts of the resulting complex variable preserve the same real physical parameters. This approach is not universally-applicable and can only be used with problems and applications which conform to the requirements of complex math techniques. Making a complex number entirely mathematically equivalent to a substantial physical problem is the real essence of complex DSP. Like complex Fourier transforms, complex DSP transforms show the fundamental nature of complex DSP and such complex techniques often increase the power of basic DSP methods. The development and application of complex DSP are only just beginning to increase and for this reason some researchers have named it theoretical DSP. It is evident that complex DSP is more complicated than real DSP. Complex DSP transforms are highly theoretical and mathematical; to use them efficiently and professionally requires a large amount of mathematics study and practical experience. Complex math makes the mathematical expressions used in DSP more compact and solves the problems which real math cannot deal with. Complex DSP techniques can complement our understanding of how physical systems perform but to achieve this, we are faced with the necessity of dealing with extensive sophisticated mathematics. For DSP professionals there comes a point at which they have no real choice since the study of complex number mathematics is the foundation of DSP.

Decoding Ogg Vorbis Audio with The C6416 DSP, using a custom made MDCT core on FPGA
Ogg Vorbis is a fairly new and growing audio format, often used for online distribution of music and internet radio stations for streaming audio. It is considered to be better than MP3 in both quality and compression and in the same league as for example AAC. In contrast with many other formats, like MP3 and AAC, Ogg Vorbis is patent and royalty free. The purpose of this thesis project was to investigate how the C6416 DSP processor and a Stratix II FPGA could be connected to each other and work together as co-processors and using an Ogg Vorbis decoder as implementation example. A fixed-point decoder called Tremor (developed by Xiph.Org the creator of the Vorbis I specification), has been ported to the DSP processor and an Ogg Vorbis player has been developed. Tremor was profiled before performing the software / hardware partitioning to decide what parts of the source code of Tremor that should be implemented in the FPGA to off-load and accelerate the DSP.
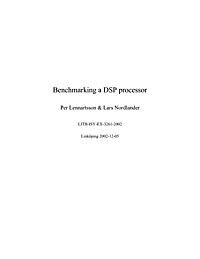
Benchmarking a DSP processor
This Master thesis describes the benchmarking of a DSP processor. Benchmarking means measuring the performance in some way. In this report, we have focused on the number of instruction cycles needed to execute certain algorithms. The algorithms we have used in the benchmark are all very common in signal processing today. The results we have reached in this thesis have been compared to benchmarks for other processors, performed by Berkeley Design Technology, Inc. The algorithms were programmed in assembly code and then executed on the instruction set simulator. After that, we proposed changes to the instruction set, with the aim to reduce the execution time for the algorithms. The results from the benchmark show that our processor is at the same level as the ones tested by BDTI. Probably would a more experienced programmer be able to reduce the cycle count even more, especially for some of the more complex benchmarks.
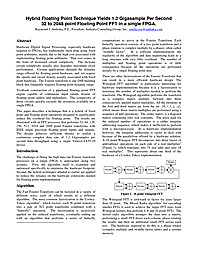
Hybrid Floating Point Technique Yields 1.2 Gigasample Per Second 32 to 2048 point Floating Point FFT in a single FPGA
Hardware Digital Signal Processing, especially hardware targeted to FPGAs, has traditionally been done using fixed point arithmetic, mainly due to the high cost associated with implementing floating point arithmetic. That cost comes in the form of increased circuit complexity. The increase circuit complexity usually also degrades maximum clock performance. Certain applications demand the dynamic range offered by floating point hardware, and yet require the speeds and circuit density usually associated with fixed point hardware. The Fourier transform is one DSP building block that frequently requires floating point dynamic range. Textbook construction of a pipelined floating point FFT engine capable of continuous input entails dozens of floating point adders and multipliers. The complexity of those circuits quickly exceeds the resources available on a single FPGA. This paper describes a technique that is a hybrid of fixed point and floating point operations designed to significantly reduce the overhead for floating point. The results are illustrated with an FFT processor that performs 32, 64, 128, 256, 512, 1024 and 2048 point Fourier transforms with IEEE single precision floating point inputs and outputs. The design achieves sufficient density to realize a continuous complex data rate of 1.2 Gigasamples per second data throughput using a single Virtex4-SX55-10 device.
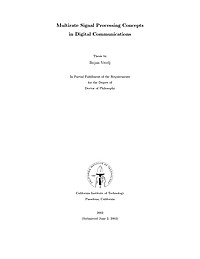
Multirate Signal Processing Concepts in Digital Communications
Multirate systems are building blocks commonly used in digital signal processing (DSP). Their function is to alter the rate of the discrete-time signals, by adding or deleting a portion of the signal samples. They are essential in various standard signal processing techniques such as signal analysis, denoising, compression and so forth. During the last decade, however, they have increasingly found applications in new and emerging areas of signal processing, as well as in several neighboring disciplines such as digital communications. The main contribution of this thesis is aimed towards a better understanding of multirate systems and their use in modern communication systems. To this end, we first study a property of linear systems appearing in certain multirate structures. This property is called biorthogonal partnership and represents a terminology introduced recently to address a need for a descriptive term for such class of filters. In the thesis we especially focus on the extensions of this simple idea to the case of vector signals (MIMO biorthogonal partners) and to accommodate for nonintegral decimation ratios (fractional biorthogonal partners). The main results developed here study the properties of biorthogonal partners, e.g., the conditions for the existence of stable and of finite impulse response (FIR) partners. In this context we develop the parameterization of FIR solutions, which makes the search for the best partner in a given application analytically tractable. This proves very useful in their central application, namely, channel equalization in digital communications with signal oversampling at the receiver. A good channel equalizer in this context is one that helps neutralize the distortion on the signal introduced by the channel propagation but not at the expense of amplifying the channel noise. In the second part of the thesis, we focus on another class of multirate systems, used at the transmitter side in order to introduce redundancy in the data stream. This redundancy generally serves to facilitate the equalization process by forcing certain structure on the transmitted signal. We first consider the transmission systems that introduce the redundancy in the form of a cyclic prefix. The examples of such systems include the discrete multitone (DMT) and the orthogonal frequency division multiplexing (OFDM) systems. We study the signal precoding in such systems, aimed at improving the performance by minimizing the noise power at the receiver. We also consider a different class of communication systems with signal redundancy, namely, the multiuser systems based on code division multiple access (CDMA). We specifically focus on the special class of CDMA systems called `a mutually orthogonal usercode receiver' (AMOUR). We show how to find the best equalizer from the class of zero-forcing solutions in such systems, and then increase the size of this class by employing alternative sampling strategies at the receiver.

Ignal Enhancement Using Time-Frequency Based Denoising
This thesis investigates and compares time and wavelet-domain denoising techniques where received signals contain broadband noise. We consider how time and wavelet-domain denoising schemes and their combinations compare in the mean squared error sense. This work applies Wiener prediction and Median filtering as they do not require any prior signal knowledge. In the wavelet-domain we use soft or hard thresholding on the detail coefficients. In addition, we explore the effect of these wavelet-domain thresholding techniques on the coefficients associated with cycle-spinning and the newly proposed recursive cycle-spinning scheme. Finally, we note that thresholding does not make an attempt to de-noise coefficients that remain after thresholding; therefore we apply time domain techniques to the remaining detail coefficients from the first level of decomposition in an attempt to de-noise them further prior to reconstruction. This thesis applies and compares these techniques using a mean squared error criterion to identify the best performing in a robust test signal environment. We find that soft thresholding with Stein’s Unbiased Risk Estimate (SURE) thresholding produces the best mean squared error results in each test case and that the addition of Wiener prediction to the first level of decomposition coefficients leads to a slightly enhanced performance. Finally, we illustrate the effects of denoising algorithms on longer data segments.

A Nonlinear Stein Based Estimator for Multichannel Image Denoising
The use of multicomponent images has become widespread with the improvement of multisensor systems having increased spatial and spectral resolutions. However, the observed images are often corrupted by an additive Gaussian noise. In this paper, we are interested in multichannel image denoising based on a multiscale representation of the images. A multivariate statistical approach is adopted to take into account both the spatial and the inter-component correlations existing between the different wavelet subbands. More precisely, we propose a new parametric nonlinear estimator which generalizes many reported denoising methods. The derivation of the optimal parameters is achieved by applying Stein’s principle in the multivariate case. Experiments performed on multispectral remote sensing images clearly indicate that our method outperforms conventional wavelet denoising techniques.
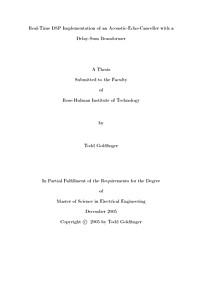
Real-Time DSP Implementation of an Acoustic-Echo-Canceller with a Delay-Sum Beamformer
Traditional telephony uses only a single receiver for speech acquisition. If the speaker is standing away from the telephone, the signal will be weak and there will be interference sources from room reverberation. In addition, there is acoustic echo coming from the loudspeaker, which further interferes with the signal of interest. This research investigated the combination of common solutions to these problems. Electronic beamforming steered an array of microphones within software to enhance the signal power. Echo cancellation removed the echo coming from the loudspeaker. In combination these processing techniques can greatly enhance user experience.
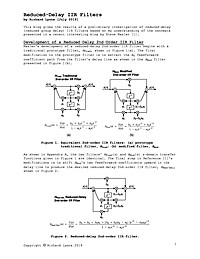
Reduced-Delay IIR Filters
This document describes a straightforward method to significantly reduce the number of necessary multiplies per input sample of traditional IIR lowpass and highpass digital filters.
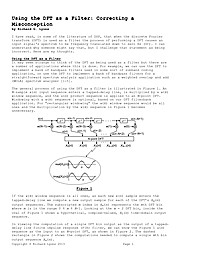
Using the DFT as a Filter: Correcting a Misconception
I have read, in some of the literature of DSP, that when the discrete Fourier transform (DFT) is used as a filter the process of performing a DFT causes an input signal's spectrum to be frequency translated down to zero Hz (DC). I can understand why someone might say that, but I challenge that statement as being incorrect. Here are my thoughts.
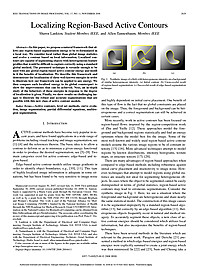
Region based Active Contour Segmentation
In this paper, we propose a natural framework that allows any region-based segmentation energy to be re-formulated in a local way. We consider local rather than global image statistics and evolve a contour based on local information. Localized contours are capable of segmenting objects with heterogeneous feature profiles that would be difficult to capture correctly using a standard global method. The presented technique is versatile enough to be used with any global region-based active contour energy and instill in it the benefits of localization. We describe this framework and demonstrate the localization of three well-known energies in order to illustrate how our framework can be applied to any energy. We then compare each localized energy to its global counterpart to show the improvements that can be achieved. Next, an in-depth study of the behaviors of these energies in response to the degree of localization is given. Finally, we show results on challenging images to illustrate the robust and accurate segmentations that are possible with this new class of active contour models.